Computational Psychiatry
Modelling neuropsychiatric disorders
This line of research focuses on modeling mathematically the multiscale mechanisms underlying perception and decision-making under uncertainty. We refer to several clinical contexts from the field of psychiatry, with a special emphasis on psychotic disorders. We combine data from experimental psychology, psychophysics, fMRI, pharmacogenetic modulation, multi-electrode signaling in humans and/or rodents.
Contacts
Key publications
The Circular Inference model
Our brain is constantly trying to resolve the uncertainty of the surrounding world. To do this, it makes predictions about the possible causes of the signals it receives. These inference operations require the propagation of messages through the cortical hierarchy. The circular inference model introduced by Jardri and Denève (2013), proposes that these aberrant predictions are due to an amplification of circulating messages. This model has received behavioral validation in schizophrenia (Jardri et al., 2017) and our work now aims to reinforce its neurophysiological plausibility.
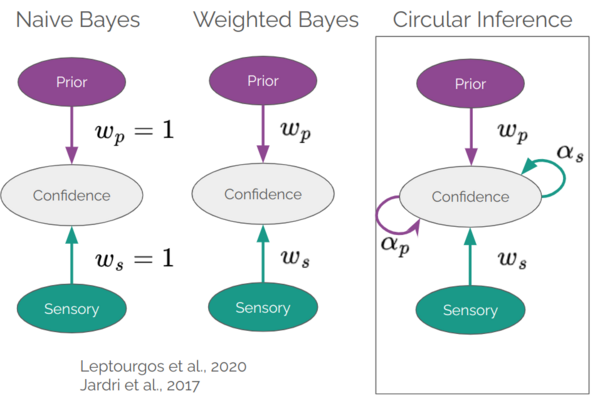
Ongoing research projects
Role of Excitation / Inhibition balance
We have seen that perception and cognition can be described in terms of Bayesian inference. From this point of view, the central nervous system, through learning and experience, captures statistics from the external world to update its internal probabilistic models and guide our behavior and actions. This research program aims to better understand how information is dynamically weighted by the brain during a decision-making task. Based on the circular inference model and a translational approach using similar tasks in rodents and humans, we are investigating the role played by the neuronal excitatory/inhibitory balance in the processing and integration of sensory information in physiological and/or pathological situations.
Spike sorting pipelines
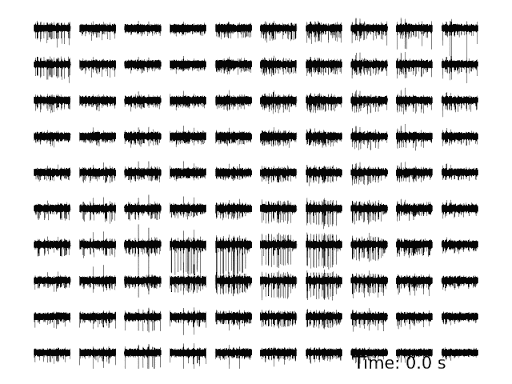
In recent years, advances in multi-electrode arrays and high-density silicon electrodes have made it possible to record extracellular signals from hundreds of electrodes. These recordings offer the possibility of reconstructing the individual activity of neurons in the vicinity of each electrode using sophisticated signal processing algorithms. However, this reconstruction remains an open problem of blind source separation (BSS), also known as “spike sorting”, which is difficult to solve due to the constraints imposed by these new devices. Firstly, the number of dimensions (i.e. electrodes) is high: algorithms have to cope with the amount of extracellular data, entering a new era of Big Data (over hundreds of Gigabytes per recording). Secondly, the density of the electrodes and the high number of sources give rise to numerous source mixtures. Most strikingly, not all existing solutions today have the capacity to operate in an online context, which is essential for advanced brain-machine interfaces (BMIs). In the team, we are actively developping new algorithms to enhance the quality of spike-sorting pipelines used worldwide both for in-vitro and in-vivo recordings.